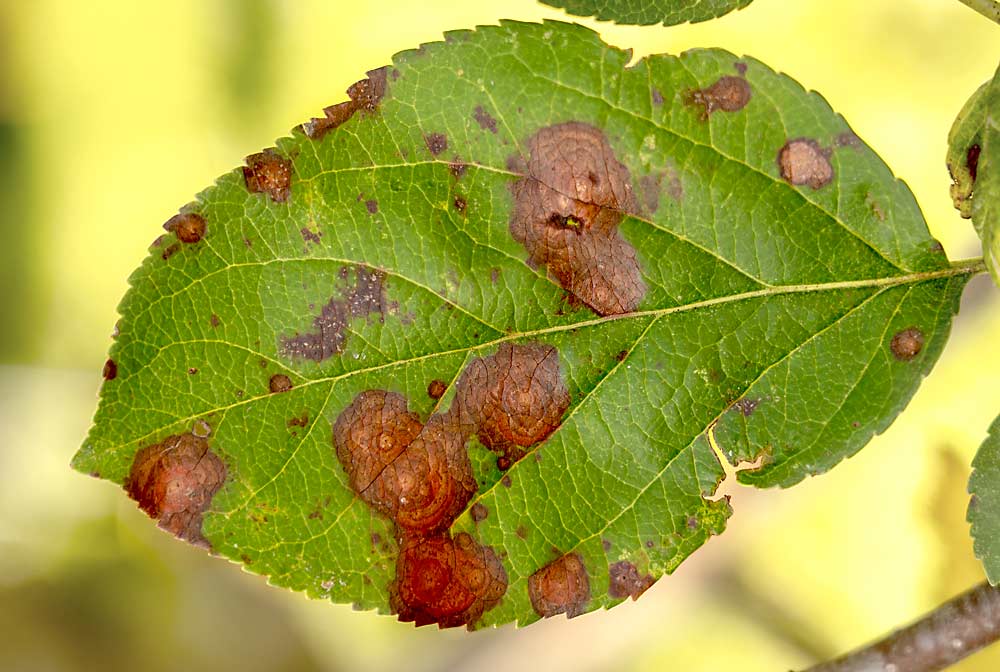
Let’s say you’re walking through your orchard, and you see spots on a leaf. It looks like a disease, but you’re not sure which one. Wouldn’t it be great if you could just take a picture of the leaf with your smartphone camera, then use an app to identify the disease?
Cornell University associate professor Awais Khan aims to produce just such an app, but first he and his team have to take a lot more pictures and do a lot more annotating.
Khan leads a project studying computer vision for rapid and accurate disease scouting in apple orchards. In the apple world, computer vision technology has gained a lot of attention for its crop load management potential, but Khan also saw potential for disease identification.
In 2019, Khan and collaborators from Cornell Tech, the university’s technology, business, law and design campus in Manhattan, received funding from the Cornell Institute for Digital Agriculture to “train” computer vision models to detect and identify apple foliar diseases, with the long-term goal of developing a system for automatic disease identification.
The first task was to collect images of apple foliar diseases. Khan’s team started with a handful of economically significant diseases, including apple scab, cedar apple rust and frogeye leaf spot. In order to properly train machine learning models, they took thousands of images of disease symptoms on leaves in Cornell research orchards. They photographed the leaves in sunny and cloudy weather, from different distances and angles, using different cameras and different focuses. They also asked growers, extension educators and crop consultants to send them images of disease symptoms.
The next task was what Khan called “expert annotation.” He and other plant pathologists used their expertise to identify the diseases in each image. Each disease must be correctly identified, because if the images are not reliable, the machine learning models will not be reliable, either, he said.
Correct identification isn’t always easy. Leaves can have multiple disease symptoms that are hard to separate. Even insect damage can look like disease damage.
“Sometimes, even experts couldn’t tell what certain symptoms were,” Khan said.
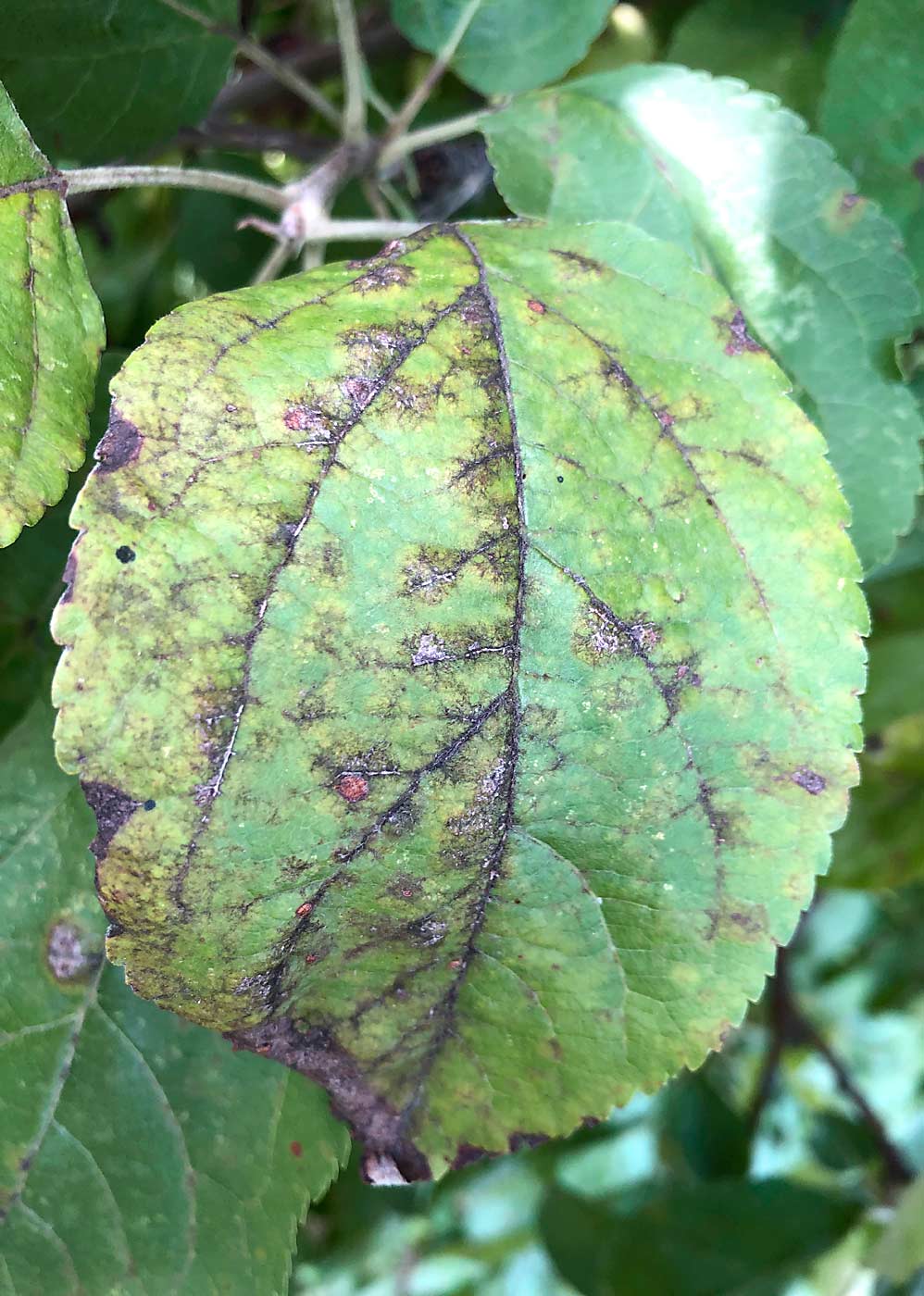
To develop the actual machine learning models, Khan and his collaborators decided to adopt a “crowdsourcing” approach. In 2020, they set up a competition on the Kaggle website (kaggle.com), a hub of coding and analytical tools shared by data scientists. Khan asked the site’s community of users to use the annotated images of apple scab, cedar apple rust and healthy leaves to develop machine learning models that could identify those diseases. Hundreds of teams from all over the world submitted thousands of machine learning models. The top teams created models that were about 97 percent accurate, Khan said.
In 2021, Khan’s team set up another Kaggle competition, submitting more complex images with a greater number of diseases, as well as multiple diseases per leaf. The resulting models weren’t as accurate. Khan’s team will continue to add images to create a larger, more comprehensive data set for future Kaggle competitions and will continue to explore more advanced methods for disease classification and quantification.
The high participation in the Kaggle competitions showed that there are many image processing and machine learning experts who can offer innovative solutions to plant disease classification. The unavailability of annotated disease data sets now serves as the main limitation, Khan said.
Developing a disease-detection app that anyone can use is one of the long-term goals of the project, but that will require years of research. In the meantime, Khan and his team are still taking pictures and making annotations.
—by Matt Milkovich
Leave A Comment