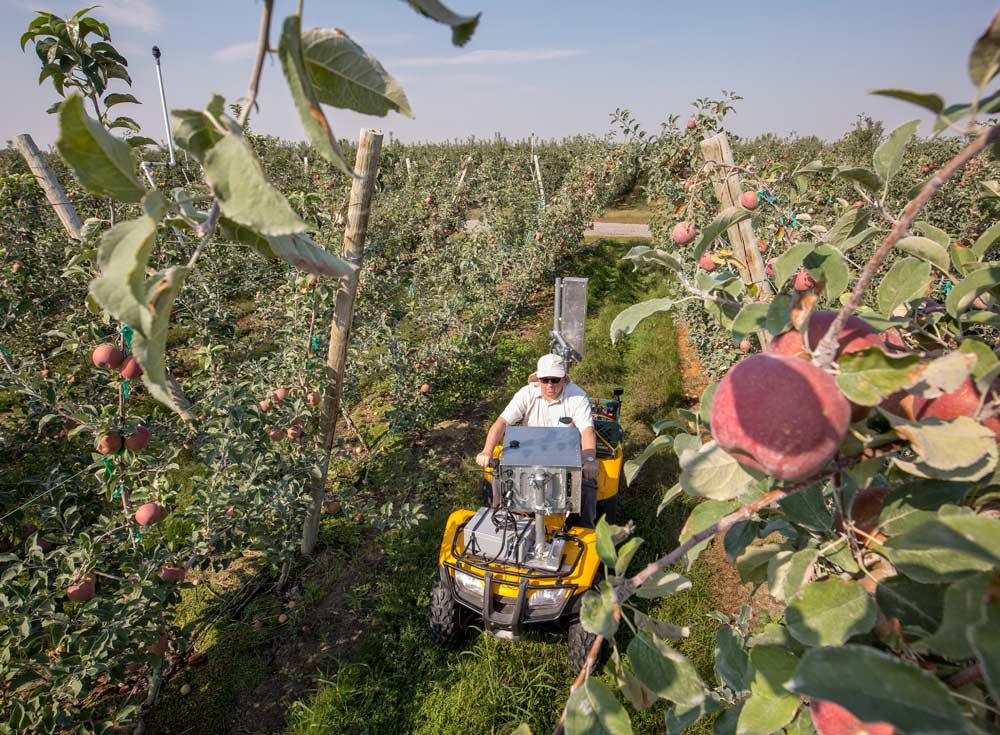
Kurt Scudder, FarmCloud imaging scientist, drives down a row of Fuji apples on an ATV loaded with camera equipment used to measure crop loads in Washington’s Columbia Basin before harvest in September. The equipment, developed by Intelligent Fruit Vision, was being evaluated by FarmCloud, which hopes to provide it as a service to growers in the Pacific Northwest. (TJ Mullinax/Good Fruit Grower)
To the casual observer, the row after row of ripe, red apples on trellises looks so uniform, it’s hard to grasp how widely production can vary from tree to tree.
In row crops, tools that enable growers to monitor yields have ushered in an enviable era of precision management, but in the tree fruit industry, hand-harvest has long made such data gathering impractical, if not impossible.
Enter the promise of technology — camera and computer systems meticulously counting apples at the fastest pace processing power will allow — to truly “see” the variability in yield at an orchard scale and turn it into useful data.
It’s a promise unfulfilled for decades, but advances in horticulture systems, computer learning and processing have resulted in new solutions that are being tested in orchards, ranging from state-of-the-art systems with real-time data crunching to smartphone snapshots for orchard sampling.
If you want to be an early adopter of this technology, it’s going to cost you.
The first commercial system available in the U.S., Intelligent Fruit Vision, sells for $40,000, plus a $6,000 annual service charge for software upgrades and maintenance.
Designed in the United Kingdom, the system, called FruitVision, uses two cameras mounted on a quad or tractor to film the canopy and count some 500,000 apples an hour as it runs calculations in real time, producing estimates of apples per tree and row, complete with size classes, and a heat map that shows the variability in production for the whole block.
It’s a very promising system, but it still has some hiccups to work out, according to one grower using it.
Other engineers are taking different approaches to try to find a lower-cost solution, by building software that can analyze images collected by smartphone or basic video camera, but those systems are still several years away from growers’ hands.
Even with the technological hurdles, engineers say computer vision technology will soon provide growers options for gathering data on fruit maturity, color development, overall yield and yield mapping.
But one system is unlikely to do all those things well, said engineer Stephen Nuske, who worked on a variety of vision systems for orchards and vineyards while at Carnegie Mellon University. Color development and maps of relative yield are technologically easier than overall yield.
That’s because calculating the size or weight of fruit is more difficult and requires far more precise resolution than simply counting apples or grape clusters, he said.
“The area I think is the most promising is mapping yields, getting the spatial data. That’s a technical gap since it’s something that didn’t exist before,” Nuske said. “Growers manage 10 fields the same, but if they knew about differences and they had tools to manage it differently, they would.”
Computer vision
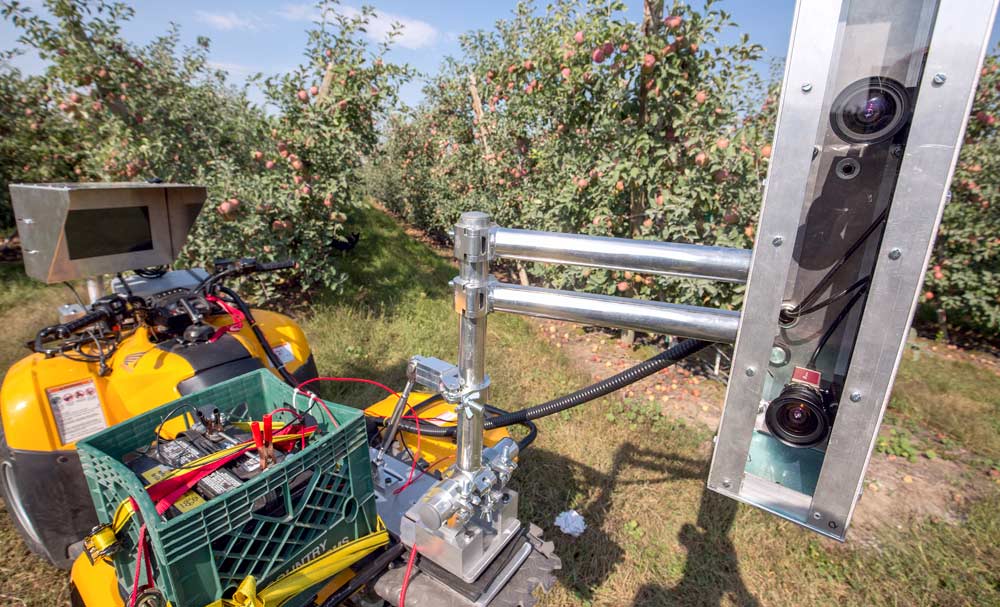
The Intelligent Fruit Vision system, called FruitVision, consists of two cameras mounted together in fixed positions so that the recorded images are in stereo, along with a powerful computer to process the images in real time. Results are displayed on the screen, which also tells the driver how fast or slow to travel through the orchard so the analysis can keep up with the images. (TJ Mullinax/Good Fruit Grower)
At the heart of the effort lies the tricky task of teaching a computer program to recognize apples on a tree, even those partially hidden by leaves or varied in color. Our human minds are so good at this it’s easy to take the complexity facing the computer for granted.
“People underestimate the difficulty of the problem. As the sun moves and shadows get cast and objects occlude each other, it’s a whole different story,” said Volkan Isler, a professor of computer science at the University of Minnesota who is working on crop estimation software in collaboration with apple breeder and horticulturist Jim Luby and graduate student Patrick Plonski.
The software also has to calculate the location of each piece of fruit in space, to prevent double counting or counting an apple that hangs in the row behind. This geometric analysis takes the most computing power, Isler said.
Growers’ ability to use this type of technology will also depend on their orchard training systems.
“The main principle is that it has to see the fruit to count or measure it,” Luby said. “In a well-managed fruiting wall, this technology is going to be able to see almost every apple. In a central leader kind of system on M.9 or M.26, it might be 40 percent you can’t see.”
So the technology needs “ground-truthing”: people counting enough trees to generate a correlation between what the cameras can detect and what’s really there, Luby said.
For its system, Intelligent Fruit Vision recommends hand counting a minimum of five trees in the first row to teach the computer program how many apples in the canopy it can’t see. The computer program then factors those “missing” apples into its estimates.
“There is no average apple tree,” said Laurence Dingle, lead inventor of the Intelligent Fruit Vision system. “This is changing how people perceive what is in their orchards.”
In Washington orchards
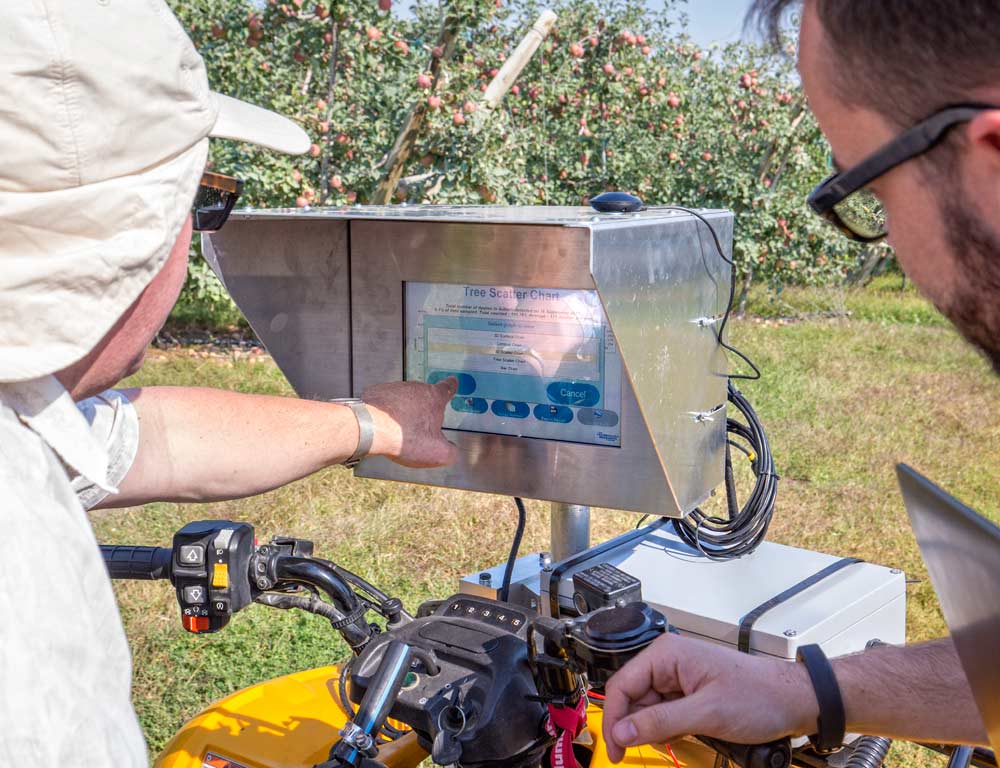
Kurt Scudder, left, and Sam Dingle use the touch monitor to control a PC that operates Intelligent Fruit Vision’s cameras, recommends speed rates to drivers and provides crop load data results in the field. (TJ Mullinax/Good Fruit Grower)
In September, the Intelligent Fruit Vision system ran slow laps in a V-trellised Fuji block in the Columbia Basin, estimating the crop load at about 171 apples per tree, with individual trees ranging from fewer than 75 to more than 200.
“In a modern, 2-D canopy like this with red apples, we’re confident we can get within a 5 percent margin of error,” said Sam Dingle, commercial administrator for Intelligent Fruit Vision. The company is a partnership between The Technology Research Centre and Worldwide Fruit, Ltd., a large U.K.-based fruit wholesaler, and the system has been in development for about five years.
The benefit of FruitVision is that it can see trends and variability in the orchard that we can’t see and turn it into data we can use, said Hermann Thoennissen, a longtime orchardist who now works as a consultant based in south-central Washington. “Everything we do in the ag world is about data now. The more data you have, the better you can plan, execute and control costs,” he said.
Yield maps like those from FruitVision are the missing piece in growers’ precision agriculture toolbox, Thoennissen said. That’s why FarmCloud, a Seattle-based ag imaging and data company he consults with, bought a system in September.
The company plans to offer it as a service to growers to complement aerial imaging and other mapping tools, said FarmCloud CEO Dennis Healy. In addition to preharvest crop load estimates that will be beneficial for sales, he expects the technology will help growers manage orchards more efficiently and someday even guide thinning.
“In the future, this technology won’t look like this. It is going to be something we can put on harvest platforms and pruning platforms to constantly gather data.”
In coming years, Intelligent Fruit Vision intends to further refine its algorithms to detect fruit blemishes and even to estimate bloom to guide chemical thinning, Laurence Dingle said.
As of 2017, Intelligent Fruit Vision has sold almost a dozen systems, mostly in Europe. Two independent tests of the technology, by the Moriniere Research Station in France and The Greenery, the marketing arm of a Dutch growers’ cooperative, confirmed that the results are consistent and quite accurate.
However, it does have limitations. Earlier in the season, when fruit is green and harder to detect, the system is not as accurate, and it’s not well suited to Granny Smith apples and green pears.
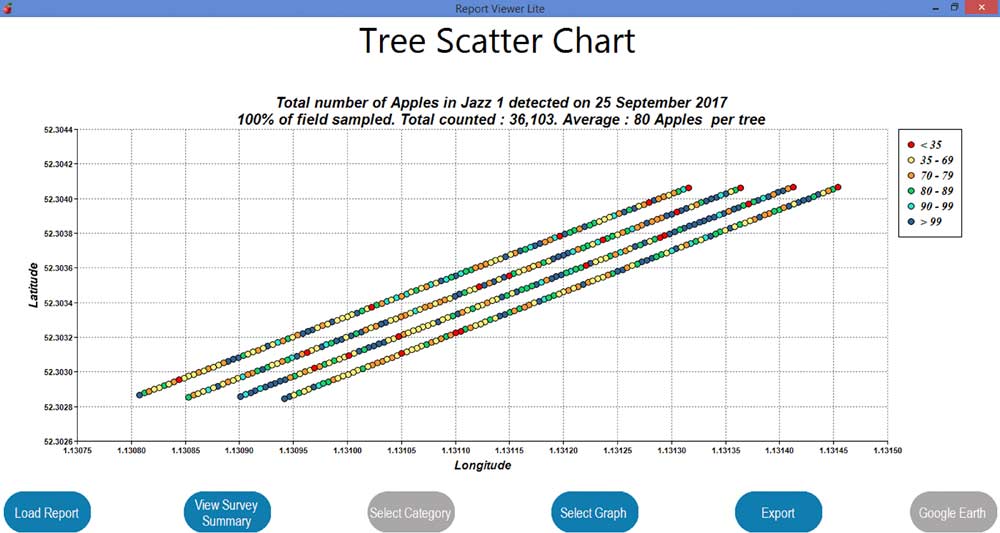
This image of the screen shows how the Intelligent Fruit Vision system presents data. In this instance, the screen displays four rows of trees and maps the variability of apples per tree detected in a Jazz orchard in the United Kingdom. Fruit per tree ranged from less than 35 apples to more than 100. The buttons at the bottom show how the data can be exported for later viewing in the office. (Courtesy of Intelligent Fruit Vision)
New York grower Rod Farrow also bought a FruitVision system this summer and used it this fall to collect crop load data on his SweetTango, Gala and Honeycrisp. He said there have been some issues with the sizing aspect of the software that the company is working to solve, but the fruit count data looks good.
“For me, the value was never in the sizing, it’s in the fruit count, because as a grower, that’s where I can make the money,” Farrow said, adding that the sizing feature definitely benefits sales and marketing. “I’m really excited about its potential.”
Can a smartphone do it?
Since it’s expensive to bring a high-powered computer to orchards, the lower cost systems in development would require transferring the image data for processing elsewhere, delaying the results somewhat.
“It’s a lot of data to crunch. So growers will send us the video. It’s more efficient to do the processing in a modern data center,” said Patrick Plonski with the UMN team. He said the goal is to build the algorithms powerful enough that it can analyze relatively low-quality video from a smartphone or other consumer camera.
After initial experiments with a complex, over-the-row system carrying several cameras to get multiple views of the canopy, Washington State University engineering professor Manoj Karkee is also narrowing his approach to images that could be collected by a smartphone that has two forward facing cameras, like some new models. “The accuracy would not be as high as the over-the-row system, but we can provide useful information to growers at almost no cost,” he said.
But it wouldn’t just be snapping a photo. For the user, it’s more likely to feel like scanning the canopy for a panoramic photo, while the device is actually collecting multiple images, Karkee said. Overlapping images are necessary so that the software can use that stereovision to estimate distance and locate each apple in three-dimensional space.
Karkee is also pursuing more of a sampling approach, imaging a subset of an orchard rather than every tree.
“Growers won’t be able to scan every tree in their 20-acre orchard. You can scan a few thousand trees and then estimate for your entire operation with calibration and extrapolation,” he said. The key to making a sampling system work is to make sure that the sampling is guided by other measures of orchard variability — soils, slopes, canopy imagery — so that the scanned trees represent an accurate snapshot of the orchard, Karkee said.
Intelligent Fruit Vision designed its system with scanning every row in mind, but, even with the automated system, it would be time-consuming in the vast orchards of the Pacific Northwest.
Top speeds are about the same as you’d probably walk so that the computer processor can keep up with the collected images.
Thoennissen and Healy said they want to test out if scanning every third or fourth row could provide sufficient data. More importantly, they want to work with growers to figure out how best to use the data that Fruit Vision and other emerging imaging technology can provide.
“Machine learning is coming. The question is how much and how soon can we integrate it into our agricultural systems,” Thoennissen said. •
– by Kate Prengaman
Leave A Comment