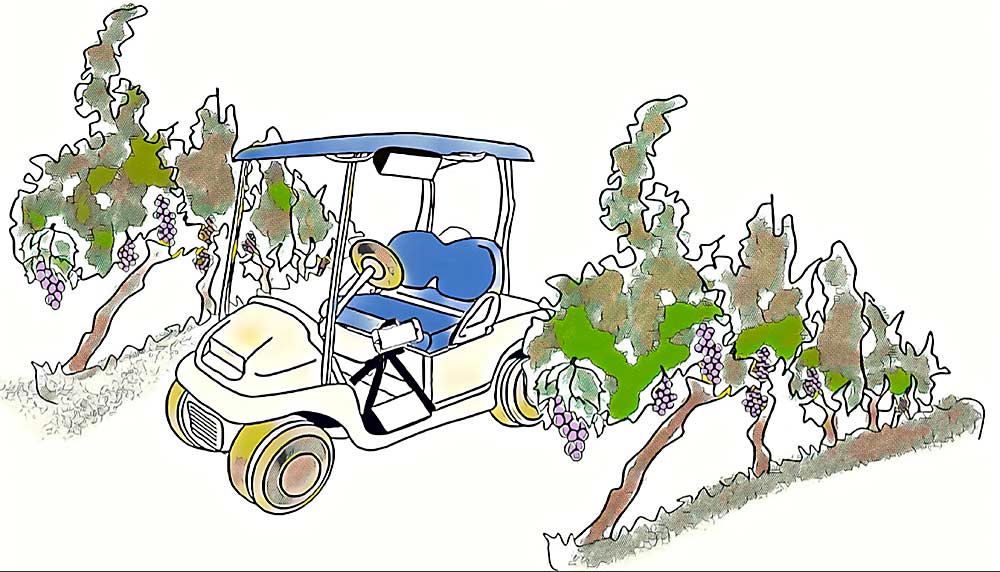
Estimating vineyard yields is not easy. You can pay people to count clusters in a subsection of your vineyard, then scale up that number to cover all your vines. But that manual method is expensive, laborious and notoriously inaccurate. You can use one of the large, sophisticated vineyard-scanning camera systems that are becoming available — the ones that take images of grapes and use them to estimate crop loads. But that’s an expensive solution for a small or medium-size grower.
What grape growers say they want is a crop estimation method that’s accurate, inexpensive and easy to use. Such as: Attach a smartphone to a tractor or ATV, drive down a row, shoot video or photographs of the clusters, and have an app use those images to estimate yields.
Researchers at Cornell University and Washington State University have been studying that approach in separate projects, and both developed smartphone apps that should be commercially available within the next few years.
The need for accurate yield estimates is crucial. After a 2016 drought, for example, some New York grape growers harvested a crop that was 30 percent larger than their original estimates. They had a hard time finding a home for all of that unexpected fruit, said Cornell plant sciences professor Justine Vanden Heuvel.
Most yield estimates are based on limited manual sampling, and the estimates can vary wildly, said Cornell engineering professor Kirstin Petersen.
New computer vision technologies can estimate yields with greater accuracy, and apps and smartphone cameras can put such technologies within reach of small and medium-size growers. Smartphones today are “amazingly capable,” with good cameras, high processing speeds and ample memory space, Petersen said.
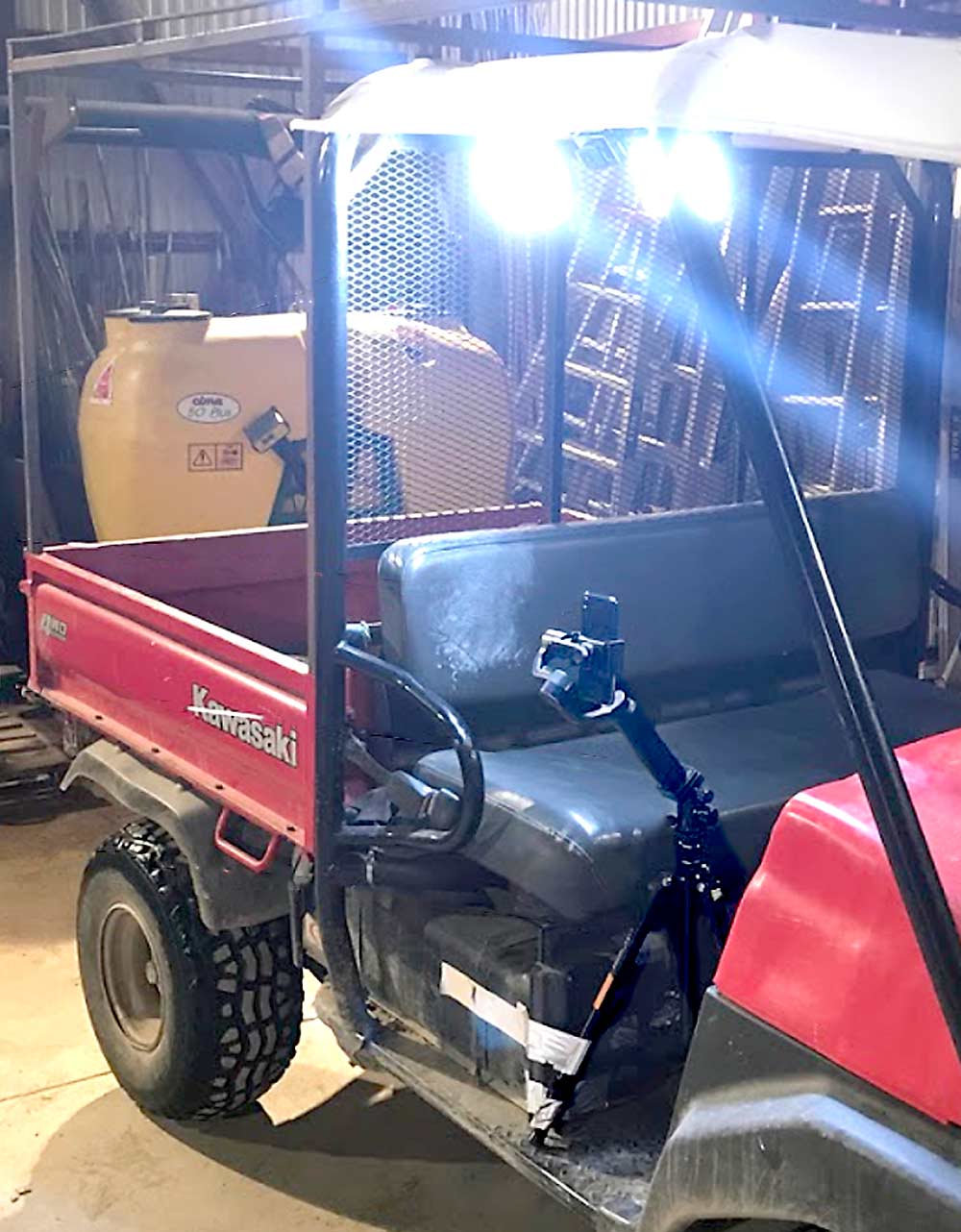
Vanden Heuvel and Petersen joined forces a few years ago to study crop estimation via smartphone and app. They received funding help from the National Science Foundation, the U.S. Department of Agriculture’s National Institute of Food and Agriculture and the Cornell Institute for Digital Agriculture. One of Petersen’s doctoral students, Jonathan Jaramillo, led the technology design.
They conduct trials at a Cornell vineyard trained to vertical shoot positioning in Lansing, New York. The team runs the trials at night, when the lack of natural light makes the lighting more even. The operator drives down a vineyard row, shining a light on the vines, while the camera records clusters. The recording is uploaded to a server that processes the data and provides a cluster count estimate.
Petersen said recordings could be made during the day if necessary, but there is less risk of making errors at night because the camera can more easily filter out objects that clutter the background.
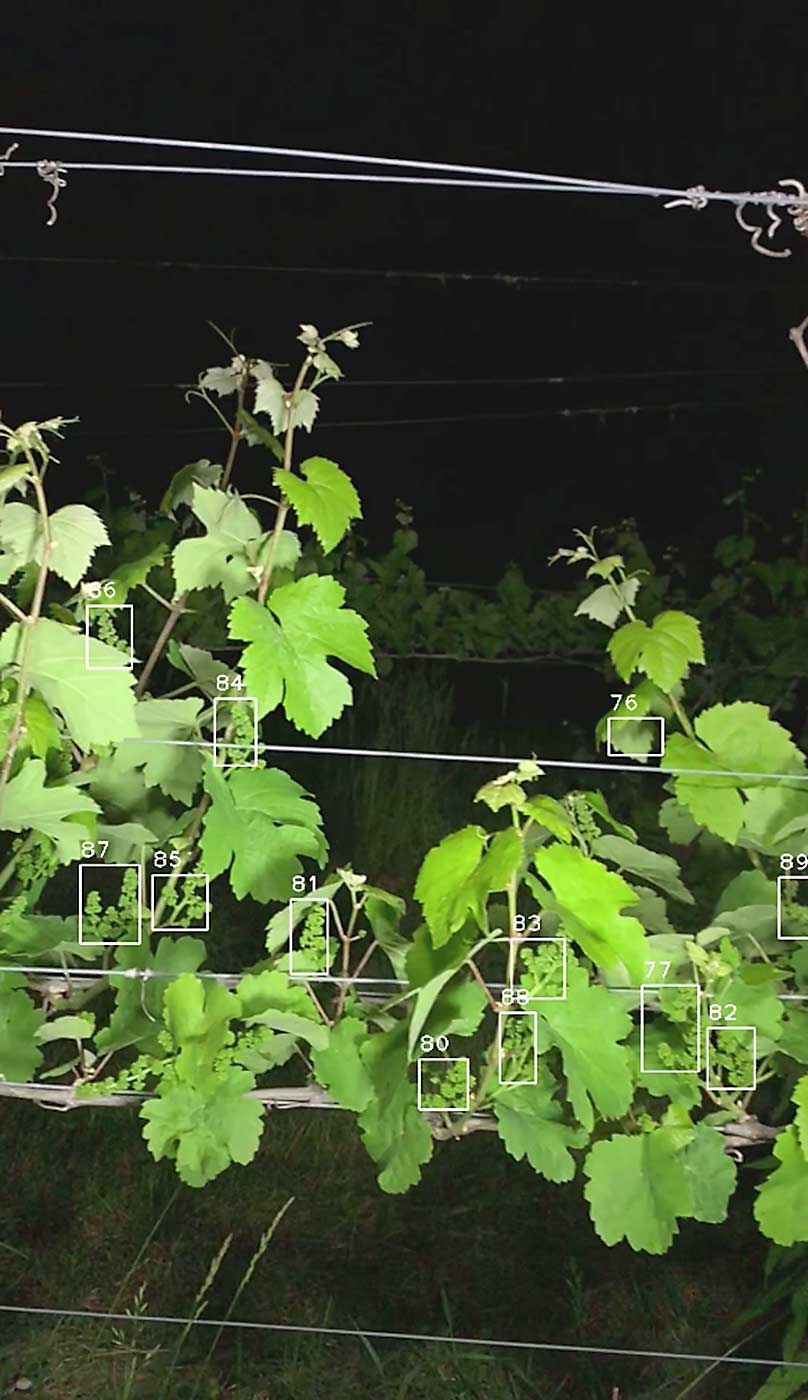
According to their results, the smartphone method achieved an average cluster count error of 4.9 percent across two growing seasons and two cultivars, compared to the manual method’s average cluster count error of 7.9 percent across the same dataset.
Another advantage of the smartphone method is that it can be used before flowering, while the canopy is sparse, which improves visibility and gives earlier yield estimates than traditional methods. Once past bloom, some clusters can be occluded by leaves or longer shoots and are harder to spot as the season progresses, Vanden Heuvel said.
After multiple seasons of testing, the Cornell researchers know they have accessible technology capable of making accurate estimates. They will field test their app this summer and hope to see it become commercially available in the next year or so, Vanden Heuvel said.
With funding from the Washington State Wine Commission’s grape and wine research program, a team of researchers led by WSU professor Manoj Karkee also studies vineyard yield estimation via smartphone camera and app in the Pacific Northwest.
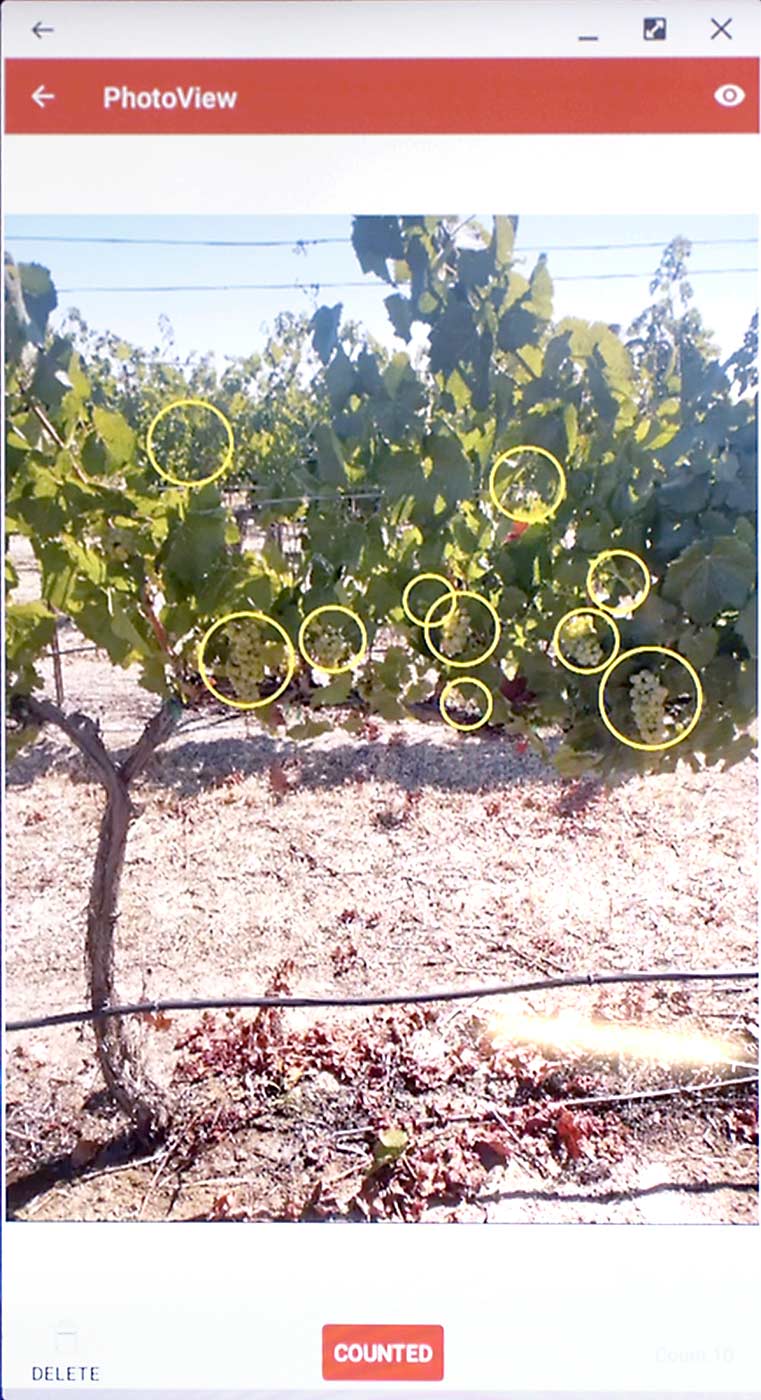
Karkee’s team has gathered data from vineyards at WSU’s Irrigated Agriculture Research and Extension Center in Prosser for a few years now and has refined their own app. They plan to test it in commercial orchards soon and hope to have a basic version in growers’ hands in the next year or so. They’re studying other potential features that could provide even more accurate estimates, such as the ability to count berries within each cluster and to estimate cluster weight from images.
Karkee also wants to expand the app’s abilities beyond crop estimation: measuring disease impact on leaves, for example, or detecting lag phase, an important physiological stage in grape growth, he said.
—by Matt Milkovich
Leave A Comment